Key to Success: Understanding Business Requirements Before Choosing a Data Modeling Technique
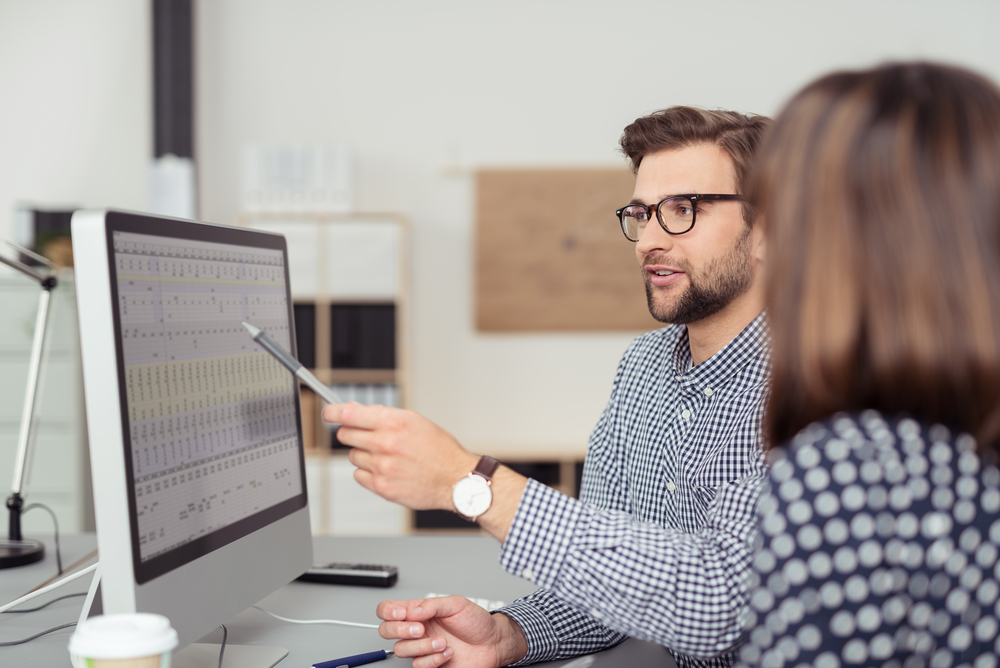
Data modeling is a crucial step in building a data warehouse, and selecting the right data modeling technique is vital to ensure the accuracy, consistency, and effectiveness of your data warehouse. However, choosing the wrong data modeling technique can lead to significant problems down the line, such as incorrect data representation, poor performance, and difficulties in maintaining and updating the data model.
To avoid these problems, it's crucial to understand your business requirements before selecting a data modeling technique.
Align data model with business needs
Your data model should accurately represent your business, including its operations, processes, and key performance indicators (KPIs). By understanding your business requirements, you can select a data modeling technique that aligns with your specific business needs. This ensures that your data model is designed to meet the specific needs of your organization, and is not simply a generic data model that may not be suitable for your business.
Prioritize data elements
Not all data is created equal, and some data elements are more important than others. By understanding your business requirements, you can prioritize data elements based on their importance to your business. This ensures that the most critical data elements are included in your data model and that they are properly represented.
Identify data sources
Understanding your business requirements also helps you identify the data sources that you need to include in your data model. This ensures that you have all the data you need to support your business operations and reporting needs.
Determine level of data granularity
Different business requirements may require different levels of data granularity. By understanding your business requirements, you can determine the appropriate level of data granularity required for your data model. This ensures that your data model includes the appropriate level of detail needed to support your reporting and analysis needs.
Improve data model accuracy and effectiveness
By aligning your data model with your business requirements, you can ensure that your data model accurately represents your business and its data. This improves the accuracy and effectiveness of your data model, which in turn improves the accuracy and effectiveness of your business operations and decision-making.
Key Take-Aways:
Understanding your business requirements is a crucial step in selecting the right data modeling technique for your data warehouse. It ensures that your data model is aligned with your business needs, includes the most critical data elements, incorporates all necessary data sources, and provides the appropriate level of data granularity. By taking the time to understand your business requirements, you can avoid common data modeling pitfalls and ensure the success of your data warehouse project.